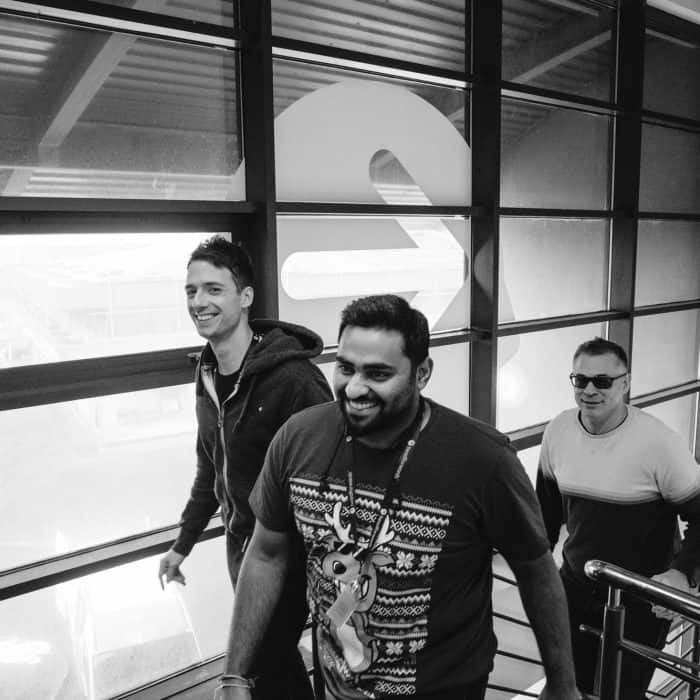
Why data quality is so important – and how you can improve yours
Data Solutions Designer Kanaga Selvi Nachiyar explains how we measure data quality and help organisations improve their data practices.
Telling stories with data is all about turning cells upon cells of data into actionable insights – useful conclusions that help leaders make smart, informed decisions.
To borrow Nate Silver’s famous analogy, data storytelling is all about finding the signal among the noise; the connections between different factors that give organisations the evidence they need to create new products, improve services, support staff, save on costs, become more profitable… Everything an organisation needs is hidden in the data somewhere.
Over the years I’ve spent as a data analyst, I’ve developed some principles that I stick to which make data analysis more effective. So, here are my seven ‘dos and don’ts’ of data storytelling:
1. Do: Get to know your data
The best story to tell with your data won’t always be the most obvious one. So you should spend as much time as possible familiarising yourself with the data – probably much longer than you think you need to.
Start with the basics first: means, medians, group sizes, and correlations. (Microsoft Power BI is very useful for this early exploratory work.)
Once you find something interesting, there’ll always be a temptation to drop the rest and run with it – but you should resist that as much as possible. In the words of the chess champion Emanuel Lasker: ‘When you see a good move, look for a better one.’
Anyone can find the most obvious conclusions with enough work, but our job as analysts is to dig deeper and find the really interesting connections – the links that can make a real difference. You may come back to your first finding later, but explore all the avenues available first.
2. Don’t: Tell a story that isn’t there
I’ve seen this problem a lot with consultants and external analysts, when they’re under pressure to come up with something to present to stakeholders. And, although we all understand the need to justify our work with a measurable outcome, sometimes those things are spurious, and can totally detract from the real story.
And sometimes there just isn’t a story to tell. For a whole host of reasons (inaccurate data, too-small datasets, ineffective collection methods, and so on), there might not be any meaningful conclusions to draw from the data – and very minor correlations are often little more than a coincidence. If you’re comparing the right datasets for the right reasons, there should be clear links to investigate.
And if there really isn’t anything to compare or connect? That can be an interesting story all by itself. If you went into an analysis expecting your data to support a clear conclusion and came up with nothing, it’s worth asking why and investigating further.
3. Do: Simplify your story
Just enough that your audience can understand it, and no more. There’s always a trade-off between accuracy and legibility in the way you present data, and there’s no quick way to determine how much you should trade off one for the other; it always depends on your audience.
For example, when I worked on some analysis for a council’s survey into staff working styles, I had a pretty good idea of the level of technical detail the client could easily understand. That meant I was able to delve deeper into the complexity of the data, explaining not only the most pressing problems, but the most pressing combinations of problems (though even then I knew not to push it too far, limiting the pairs of problems rather than trios).
4. Don’t: Choose a fancy algorithm over a useful one
Every model and algorithm you use in an analysis should be easy to interpret. In some cases, especially if you’re working with machine learning, that will mean choosing something a little less powerful.
Something like a general linear model is good because you can simply show a graph of inputs (such as the amount of funding) and outputs (like mental health scores). A fancy neural network might be more accurate, but it’ll be harder to understand how and why it came to its conclusions, which makes it less useful for business decisions. If your algorithm is just a black box that churns through data and spits out a conclusion, it’s difficult to point to individual factors or interactions.
5. Do: Build charts, not tables
This is a very simple principle: when you’re presenting data, charts are always better than tables.
It’s hard to interest people in tables full of numbers, and you risk not getting your points across clearly. Charts are visually intuitive – and, with the right tools, easy to produce – which makes them a quick, easy way to communicate your findings and engage stakeholders.
6. Don’t: Get distracted by the surprises
Happening upon a bombshell finding is always exciting. But the unfortunate truth is that surprising things may be interesting, but they’re also usually wrong. Be super careful if you’re examining or presenting something that sounds counterintuitive – it’s often the case that conclusions that come out of nowhere are products of faulty analysis, not a landmark finding.
This saved me from making a fool of myself more than once. For example, I almost found that more deprived areas had better mental health outcomes, before I realised that I’d accidentally reversed the axis. Always check your work.
7. Do: Know when you’ve exhausted your data
My final principle of data storytelling is, essentially, ‘know when to quit’. As I mentioned above, you should never settle for the first obvious conclusion when you’re analysing your datasets – but you do need to learn how to recognise when there’s nothing left to find.
This is a knack that comes with experience. Some datasets are richer than others, but the bottom line is that they all have a limit to what they can support (the quality of output will always depend on the skills, experience, and creativity of your analysts, too).
When you’re analysing data – or working with analysts – it all comes down to being diligent, accurate, and realistic about what you want to achieve with your data. Then, as long as you don’t get distracted by your first finding or an unexpected bombshell, you’ll have a detailed, actionable story to tell by the time your dataset is exhausted.
Headforwards™ is a Registered Trade Mark of Headforwards Solutions Ltd.
Registered Address: FibreHub, Trevenson Lane, Pool, Redruth, Cornwall, TR15 3GF, UK
Registered in England and Wales: 07576641 | VAT Registration Number: GB111315770