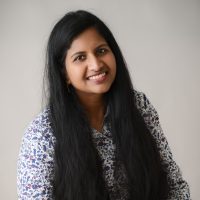
As tech users, we’re creating data all the time – even when you’re not thinking about it. We create data every time we use a piece of software, log in to a website, or download a file. Everything we do leaves a digital trail that has to go somewhere.
Within organisations, that means mountains of data to manage from their employees and end users. Data drives everything, so when data quality is low, so many business processes and outcomes are affected.
In our work with a local authority, we’ve been focusing on master data management – building a single system that consolidates all the authority’s separate records. This will create one totally accurate, detailed record for every citizen, making it easier for them to access services and ensuring council workers have everything they need to provide holistic support.
And that’s gotten me thinking about how important good quality data is for effective products and services. You may have heard the phrase “garbage in, garbage out” before. It basically means that the results of an analysis are only ever as good as the data it uses – and the same is true for other applications of data too.
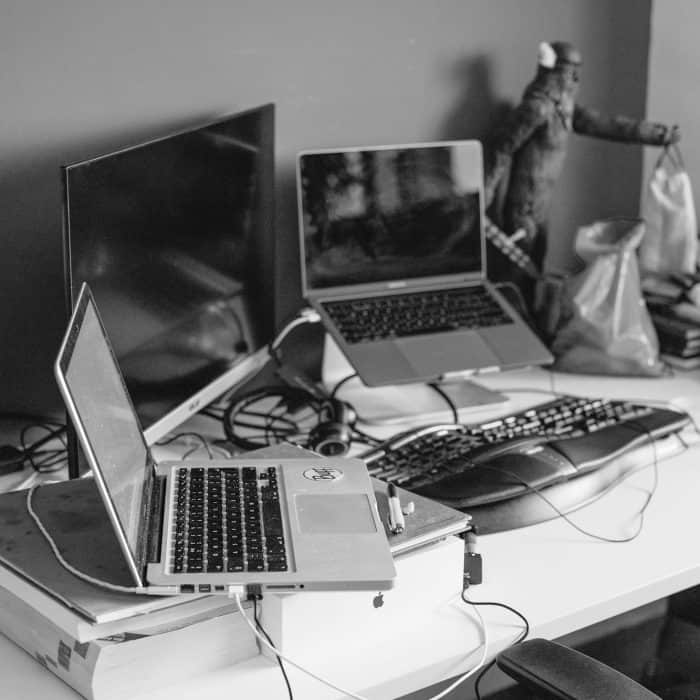
Everyone shares the responsibility for data quality
There are a lot of factors that affect data quality, from the way it’s collected to the way it’s stored – and the consequences of poor data can be significant.
Studies by Gartner have shown that bad data can cost companies as much as $15 million every year – and 60% of organisations aren’t even tracking the impact. If you have poor data practices, it’s harder to maintain customer trust and your reputation within your industry. And in some sectors, the fines and sanctions associated with lapses in data processes can be massive.
Part of the issue comes from organisations assuming that data is just the responsibility of the IT or analytics team. But we’re all responsible for the quality of the data we provide, both at work and in our personal lives.
For example, if you update your address with a retailer, you need to ensure you’re giving them the right postcode and house number if you want your package to arrive in the right place. Similarly, if you’re a salesperson inputting new customer data, you need to ensure the information is accurate, so your colleagues can use it to provide great service later on.
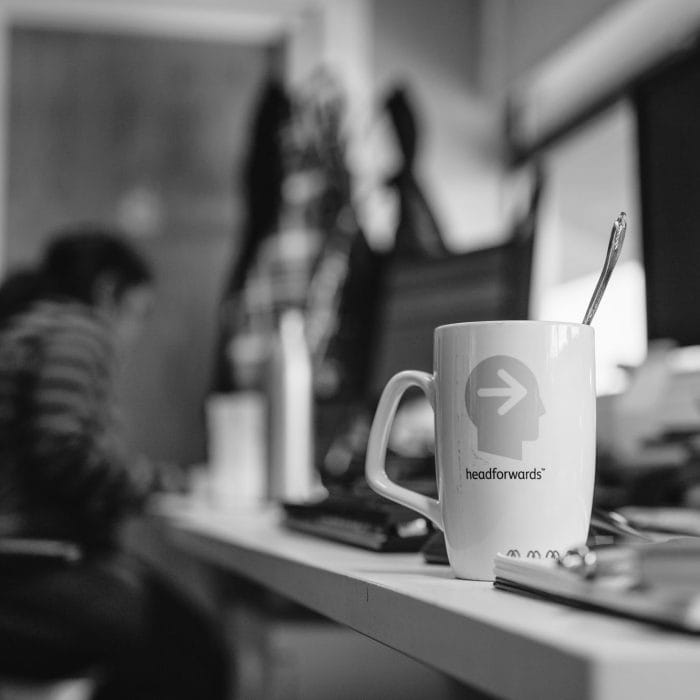
Understanding your data is the first step to improving quality
Digital transformation projects usually start with a long, hard look at an organisation’s data quality.
Data profiling is helpful as an initial assessment before we analyse data in depth – we can identify the format, whether there’s content missing, and whether it’s up to date. We can also track the data’s lineage, which gives us information about how the data’s processed, which systems use it, and what it’s used for.
There are five main measures that we use:
- Accuracy – Is this the right information?
- Completeness – Does this record have all the necessary information? (For example, providing just a postcode on an address form may be accurate, but it’s not complete.)
- Consistency – Is this record the same across multiple systems? Is it easy to reconcile data from different sources?
- Uniqueness – Is this data duplicated unnecessarily in another system?
- Timeliness – Is this information up to date? Is it easily available when we need it? (The nearly 16,000 COVID test results that went unreported due to a spreadsheet error is an excellent example of the importance of timely access to accurate data.)
This doesn’t all need to be done manually. Service providers like Informatica, Microsoft, Oracle, Salesforce, and more all offer data quality tools that can automate and accelerate this assessment process – and help set the rules that protect data quality going forward.
Simple changes can have an enormous impact
Implementing rules for data input and management across your organisation can help ensure you’re all working to the same standards of accuracy, completeness, and the other key quality metrics.
For example, something as simple as restricting phone number fields to numbers-only can stop people mistyping a zero as an “O”. Introducing automated address lookups and dropdowns instead of free text fields can ensure every customer uses the same format for their home address. And standardising dd/mm/yy for your date input can clear up confusion between, say, the 8th of June and the 6th of August.
Consolidating and connecting systems is also important for ensuring consistency and avoiding duplication across the organisation. Let’s say an employee leaves for a new job. When their status changes in the HR system, does it also change in the IT team’s systems? With better visibility of who’s on the team, IT can reassign software licences or reset their work laptop for a new user, ensuring expensive tools and equipment don’t go unused.
Prioritise accuracy to save future effort
My final advice for boosting data quality is to prioritise accuracy over speed. That can be difficult in the busy world of running an organisation, but ensuring you take the time to check the validity of your data will save you future headaches.
Human error, differences in data format, individual quirks of your core systems – there are a lot of opportunities for data quality to drop, and organisations often spend a lot of extra time and effort remediating quality issues. But by establishing organisation-wide standards and regularly profiling your data to highlight records that need attention, you can improve data quality throughout your systems – which in turn means more accurate, useful results from your analytics and other data projects.